Co-Project leader: Dr. Jozefus Schippers
Email:Staff:
- Dr. Johannes Thiel
- Dr. Markus Kuhlmann
In the frame of the joint AVATARS project, an extensive multi-omics approach will be conducted to gain a detailed picture of rapeseed seed development and germination. Analysis of omics data will allow the generation of predictive models to assist future breeding approaches in Brassica napus L.
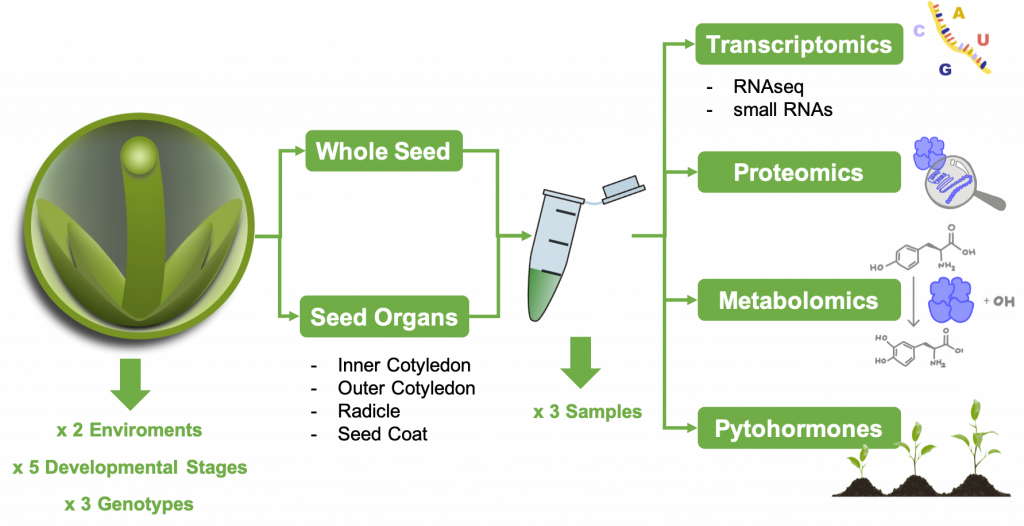
Here, seed development in three contrasting rapeseed genotypes under optimal and detrimental cultivation regimes will be analysed. To this end, seed material will be collected at three to five distinct stages of plants grown in the plant cultivation hall (PKH). To increase the spatial resolution of our analysis, the developing seeds will be dissected into inner and outer cotyledon (IC and OC), radicle (RA), and seed coat (SC). Sampled material from a common pool will be subjected to different types of downstream analyses, therefore coordination of the sampling is essential.
Samples will be used for multi-omics analyses that include transcriptomics, epigenomics, small RNA-omics, proteomics, metabolomics and analysis of plant hormones. These platforms have been established at the IPK-Gatersleben or the University of Hannover where also expertise in rapeseed development is existing. The data obtained from these analyses will gain insights into spatiotemporal changes in gene expression, protein levels, metabolites and hormones together with a change in the epigenetic landscape in seeds of different genotypes grown under both environmental conditions.
Expressed genes will be mapped onto metabolic, hormonal and regulatory pathways to support the identification of key events and processes during seed development. In addition, the expressional changes will be linked to potential post-transcriptional gene silencing or transcriptional gene silencing and RNA directed DNA methylation events. Furthermore, the correlation between transcript changes and actual protein level changes will be assessed. Subsequently, seed-specific metabolic and transcriptomic networks will be reconstructed to identify key determinants and targets and to generate predictive models for beneficial seed properties through deep learning approaches.